WIMI Hologram Academy: Two New Techniques for Phase Disentanglement
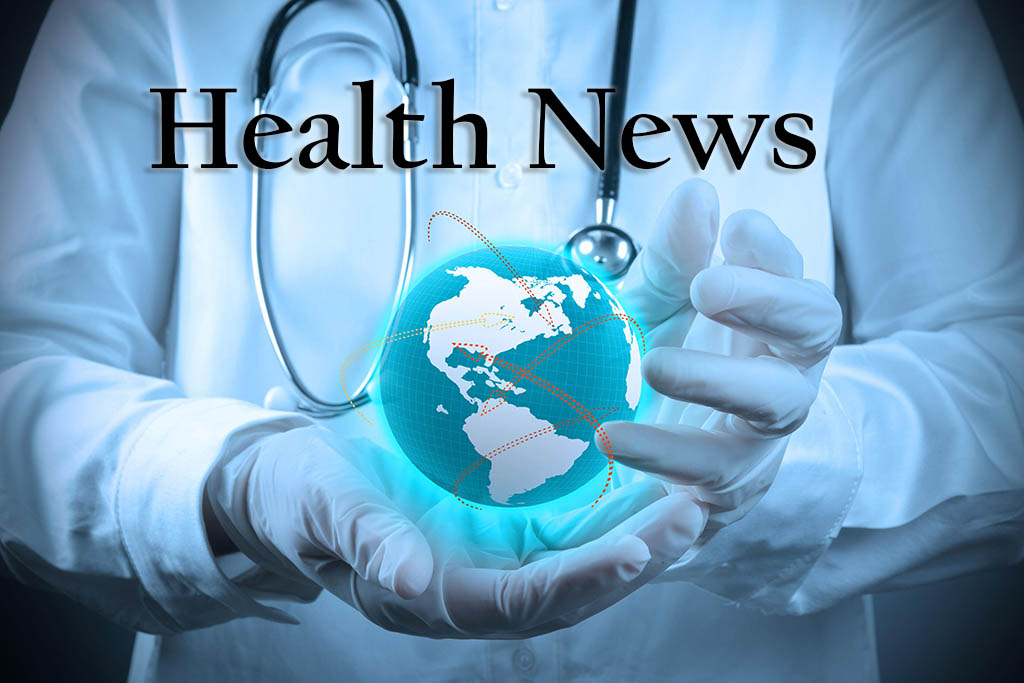
HONG KONG, Sept. 22, 2022 (GLOBE NEWSWIRE) — WIMI Hologram Academy, working in partnership with the Holographic Science Innovation Center, has written a new technical article describing their research on Two New Techniques for Phase Disentanglement. This article follows below:
Phase disentanglement is a kind of recovery from the measured winding phase (0 to 2) that can directly reflect the absolute phase of the physical characteristics of interest (Eliminate 2 Ambiguity) technology, the technology plays a very important role in interference synthetic aperture radar (InSAR), magnetic resonance imaging (MRI) and optical interferometry, can be said to be one of the core technology of these applications. Especially in the InSAR field, the quality of the phase disentanglement results directly determines the accuracy of the subsequent topographic elevation information and deformation information. To this end, a lot of research has been conducted on phase unwinding technology, among which the fastest development is that in the field of InSAR technology. Scientists from WIMI Hologram Academy of WIMI Hologram Cloud Inc.(NASDAQ: WIMI) focus on the development of phase unwinding technologies used in InSAR, focusing on two of the most advanced phase unwinding technologies.
In the 1980s, the single-baseline phase disentanglement technology was first developed rapidly, which can be divided into two categories, the first is based on path tracking, and the second is based on the minimum norm. In 1986, the United States first applied phase unwinding technology to InSAR, proposed a branch-based (Branch-Cut) method, and demonstrated the world’s first airborne InSAR winding interference phase diagram. The method detects the distribution of something called the residual handicap in the interferometric gram, by designing reasonable branch tangents to connect these handicaps, and then recovers the disentangled phase through the path integral under the principle of not crossing the branch tangent.
In the 1990s, the study of path tracking-based phase unwinding methods began to explode, making this method a very representative classic method of single-baseline phase untangling methods, including branch method, minimum discontinuity method, and minimum spanning tree method. At the same time, the second type of method has also begun to attract the attention of researchers in various countries. The main representative methods are the unweighted least squares method, the weighted least squares method, and the minimum Lp norm method. Later, advanced single-baseline winding methods of minimum cost flow (MCF), statistical cost flow (SNAPHU), PUMA, and large-scale phase unwinding techniques appeared.
Later, multi-baseline phase unwinding was slowly developed, and single-baseline phase unwinding does not need to follow the phase continuity assumption, which requires that the absolute phase difference between adjacent pixels be less than and thus can be applied to areas with dramatic topographic changes. Later, multi-baseline phase unwinding was slowly developed, and single-baseline phase unwinding does not need to follow the phase continuity assumption, which requires that the absolute phase difference between adjacent pixels be less than and thus can be applied to areas with dramatic topographic changes. Multi-baseline phase disentanglement method is also mainly divided into two categories: the first class is parameter-based on the method of the interference phase probability density function to build a statistical framework of the terrain elevation or elevation difference as an estimated parameter, and estimate the parameter according to the maximum likelihood criterion (ML) or maximum posterior criterion (MAP), to realize phase disentanglement. The second class is non-parametric-based methods which directly use absolute interference phases using unsupervised learning techniques to estimate without the need to exploit the interference phase probability density function. The following are the two most representative and advanced new techniques for multi-baseline phase untangling: Cluster Analysis and Two-Stage Programming Approach.
Cluster analysis is the first advanced method of multi-baseline phase disentangling. The method first obtains the corresponding intercept map according to the input multi-baseline interference map; then uses the statistical histogram to divide all the pixels into different classes according to the intercept size, where each class pixel has the same fuzzy number vector; Then find the central intercept of each pixel, and calculate the fuzzy number vector for each pixel according to the size of the center intercept; finally, recover the absolute phase of each pixel class by class. Compared with the traditional pixel-by-pixel multi-baseline phase unwinding algorithm, those based on cluster analysis have stronger noise robustness and higher time efficiency. The steps of the cluster analysis algorithm described above are only the basic steps.
besides, Some scholars combine multiple interferences maps to improve the distance between the center intercept of each class of pixel in the clustering algorithm, Some scholars have extended the pixel feature information needed to be used in the cluster analysis from the original one-dimensional intercept information to the three-dimensional intercept and distance direction, azimuth direction and other information, Some scholars have optimized the disadvantage of solving the pixel ambiguity vector through search in the early clustering algorithms, The corresponding closed solution formula is given, It is also pointed out that the accuracy of the disentangled phase can be further improved after completing the clustering, In addition, the robustness of multiple baseline phase disentanglement can be improved by designing the optimal baseline combination. With the above improvements, the performance of the multi-baseline phase Algorithm based on cluster analysis is greatly improved. In 2021, some scholars introduced deep learning technology into the multi-baseline phase disentanglement algorithm based on cluster analysis, successfully transforming the multi-baseline phase unwinding problem into an unsupervised cluster analysis problem. They exploit the high feature descriptive of deep learning techniques to propose an unsupervised deep convolutional neural network called CANet.This method first clusters all pixels into different classes based on the recognizable pattern of the input multi-baseline interference phase ambiguity, and then extends another advanced multi-baseline phase unwinding method to the multi-baseline phase unwinding processing on a sparse irregular network established based on the CANet clustering results. Theoretical analysis and experimental results show that the proposed method is an efficient multi-baseline phase unwinding method, with an execution time much lower than many classical multi-baseline phase unwinding methods.
The second classical multi-baseline phase unwinding method is the two-stage planning method, the idea of a single baseline phase unwinding into the multi-baseline phase unwinding, using the Chinese remainder algorithm to estimate the phase gradient between adjacent pixels in the first stage, and improve the noise robustness. The method was first proposed in 2016 and has since attracted great attention from researchers because it established a link between single-baseline and multi-baseline phase disentanglement. In 2019, an improved two-stage planning method based on the local plane model assumption was proposed, which approximates the actual terrain, thus improving the robustness of the phase gradient estimation algorithm in the first phase. At the same time, others proposed an improved two-stage planning method based on the trace-less Kalman filter, which applied the trace-free Kalman filter to the second stage of the two-stage planning method, so that the two-stage planning method has the function of phase filtering, so the performance is further improved. Some scholars also try to introduce Bayesian filtering technology into the two-stage method, established two-stage programming based Bayesian multi-baseline phase winding framework, proposed multi-baseline extension Kalman filtering (EKF) phase winding algorithm, multi-baseline volume Kalman filter (CKF) phase unwinding algorithm and multi-baseline tasteless information filtering (UIF) phase entanglement algorithm three improved versions of the two-stage programming method. In addition, some scholars have introduced the maximum flow / minimum cut algorithm (PUMA) into the two-stage planning method. In the second phase, the Markov Random Field (MRF) model is designed to model the local context correlation. Compared to the original two-stage planning method, the proposed technique greatly improves the performance of the multi-baseline phase unwinding technique in areas of dramatic topographic changes. They proposed three improved two-stage planning methods: multi-baseline Extended Kalman Filter (EKF) phase unwinding algorithm, multi-Cubage volume Kalman Filter (CKF) phase unwinding algorithm, and multi-baseline tasteless information filtering phase unwinding algorithm. In addition, some scholars have introduced the maximum flow / minimum cut algorithm (PUMA) into the two-stage planning method, in the second phase of the Markov random field (M U M F) model to model local context correlation. Compared to the original two-stage planning method, the proposed technique greatly improves the performance of the multi-baseline phase unwinding technique in areas of dramatic topographic changes.
According to the above analysis, the new technology of multi-baseline phase unwinding based on cluster analysis algorithm and two-stage planning method has a very important role in phase unwinding and must be an important basis for the further development of multi-baseline phase unwinding technology in the future.
Founded in August 2020, WIMI Hologram Academy is dedicated to holographic AI vision exploration and researches basic science and innovative technologies, driven by human vision. The Holographic Science Innovation Center, in partnership with WIMI Hologram Academy, is committed to exploring the unknown technology of holographic AI vision, attracting, gathering, and integrating relevant global resources and superior forces, promoting comprehensive innovation with scientific and technological innovation as the core, and carrying out basic science and innovative technology research.
Contacts
Holographic Science Innovation Center
Email: pr@holo-science. com